AI-Driven Threat Detection and Response
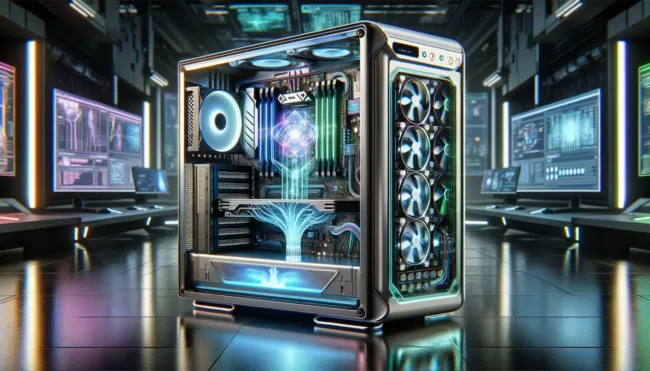
Posted on October 30, 2024 by Rob May
Welcome to our technical blog, where we share insights and expertise on a variety of technical topics. This post is part of our ongoing series, specifically aimed at professionals in technical roles, providing in-depth information and practical tips.
Cyber threats are evolving at an unprecedented pace, the ability to detect and respond to these threats in real-time is critical for maintaining robust cybersecurity. Artificial intelligence (AI) has emerged as a game-changer in this domain, offering sophisticated methods for identifying and mitigating security threats more effectively than traditional approaches. This blog will explore how AI-driven systems enhance threat detection and response, providing insights into the technologies, applications, and benefits of these advanced solutions.
The Need for AI in Threat Detection and Response
Traditional cybersecurity measures often rely on predefined rules and signatures to detect threats. While effective to some extent, these methods struggle to keep pace with the rapidly changing threat landscape. Cyber attackers continually develop new techniques to bypass conventional defences, necessitating more adaptive and intelligent security solutions.
AI-driven threat detection and response systems address this challenge by leveraging machine learning (ML), deep learning, and other AI technologies to analyse vast amounts of data, identify patterns, and respond to threats in real-time. These systems can learn from past incidents, adapt to emerging threats, and automate complex security tasks, significantly enhancing the overall security posture of an organisation.
AI Techniques in Threat Detection
Behavioural Analysis
AI-driven behavioural analysis involves monitoring and analysing the behaviour of users, devices, and applications to detect potential threats. Machine learning models can establish baselines of normal behaviour and identify deviations that may indicate compromised accounts or insider threats.
For example, if a user’s login pattern suddenly changes, such as logging in from multiple locations within a short period, the AI system can flag this as suspicious and initiate further scrutiny or automated responses, such as temporary account lockdowns.
Anomaly Detection
AI excels at detecting anomalies, which are deviations from normal behaviour that may indicate a security threat. Unsupervised learning algorithms, such as clustering and autoencoders, can identify unusual patterns in network traffic, user behaviour, and system logs without requiring labelled data.
For instance, an AI system might monitor network traffic to detect sudden spikes in data transfer rates or unusual access patterns to sensitive data. By recognising these anomalies, the system can alert security teams to potential breaches or malicious activities that require further investigation.
Pattern Recognition
Deep learning models, particularly Convolutional Neural Networks (CNNs) and Recurrent Neural Networks (RNNs), are highly effective at recognising patterns in complex data sets. These models can analyse various data sources, including network traffic, system logs, and application behaviour, to identify signatures of known threats and detect new, previously unseen threats.
For example, CNNs can be used to analyse network traffic data to identify patterns associated with specific types of cyber-attacks, such as distributed denial-of-service (DDoS) attacks. RNNs, on the other hand, are well-suited for analysing sequences of events, making them ideal for detecting patterns in log data that may indicate a security breach.
AI-Driven Response Mechanisms:
Automated Incident Response
One of the most significant advantages of AI in cybersecurity is its ability to automate incident response. By leveraging AI, organisations can reduce the time it takes to respond to security incidents, minimising the potential damage caused by breaches.
AI-driven response mechanisms can include isolating affected systems, blocking malicious IP addresses, and applying security patches automatically. For instance, if an AI system detects a ransomware attack in progress, it can immediately isolate the compromised endpoints to prevent the spread of the malware.
Adaptive Security Measures
AI systems can adapt to changing threat landscapes by continuously learning from new data and incorporating feedback from security analysts. This adaptive capability allows AI-driven security solutions to remain effective against evolving threats and minimise the need for constant manual updates.
For example, an AI-driven security system can adjust its rules and policies based on real-time threat intelligence, blocking emerging threats without requiring manual intervention. Similarly, AI-powered intrusion detection systems (IDS) can update their detection algorithms based on the latest attack vectors and techniques.
Predictive Analytics
AI-driven predictive analytics can forecast potential security incidents by analysing historical data and identifying patterns that precede attacks. By understanding these patterns, organisations can proactively strengthen their defences and mitigate risks before they materialise.
For instance, predictive analytics might reveal that a specific type of attack tends to occur following certain network activities or user behaviours. Armed with this information, security teams can implement pre-emptive measures, such as increased monitoring or additional access controls, to prevent potential breaches.
Benefits of AI-Driven Threat Detection and Response:
Improved Accuracy
AI-driven systems can analyse vast amounts of data with high accuracy, reducing the likelihood of false positives and false negatives. This precision enables security teams to focus on genuine threats rather than wasting time on benign activities.
Faster Response Times
By automating threat detection and response, AI significantly reduces the time it takes to identify and mitigate security incidents. This rapid response is crucial in minimising the impact of cyber-attacks and preventing further damage.
Enhanced Scalability
AI systems can scale to handle large volumes of data and complex security environments. This scalability is essential for organisations of all sizes, particularly those with extensive digital infrastructures and numerous endpoints to protect.
Continuous Learning and Adaptation
AI-driven security solutions continuously learn from new data and adapt to emerging threats. This ability to evolve ensures that these systems remain effective against the latest cyber threats and do not become obsolete over time.
AI-driven threat detection and response represent a significant advancement in cybersecurity, offering enhanced accuracy, faster response times, and the ability to adapt to evolving threats. By leveraging AI technologies, organisations can improve their security posture and better protect their digital assets in an increasingly hostile cyber landscape.
As we continue this series, our next blog will delve into the role of natural language processing (NLP) in cybersecurity, exploring how NLP technologies are used to detect phishing attempts, analyse sentiment, and identify malicious communications.
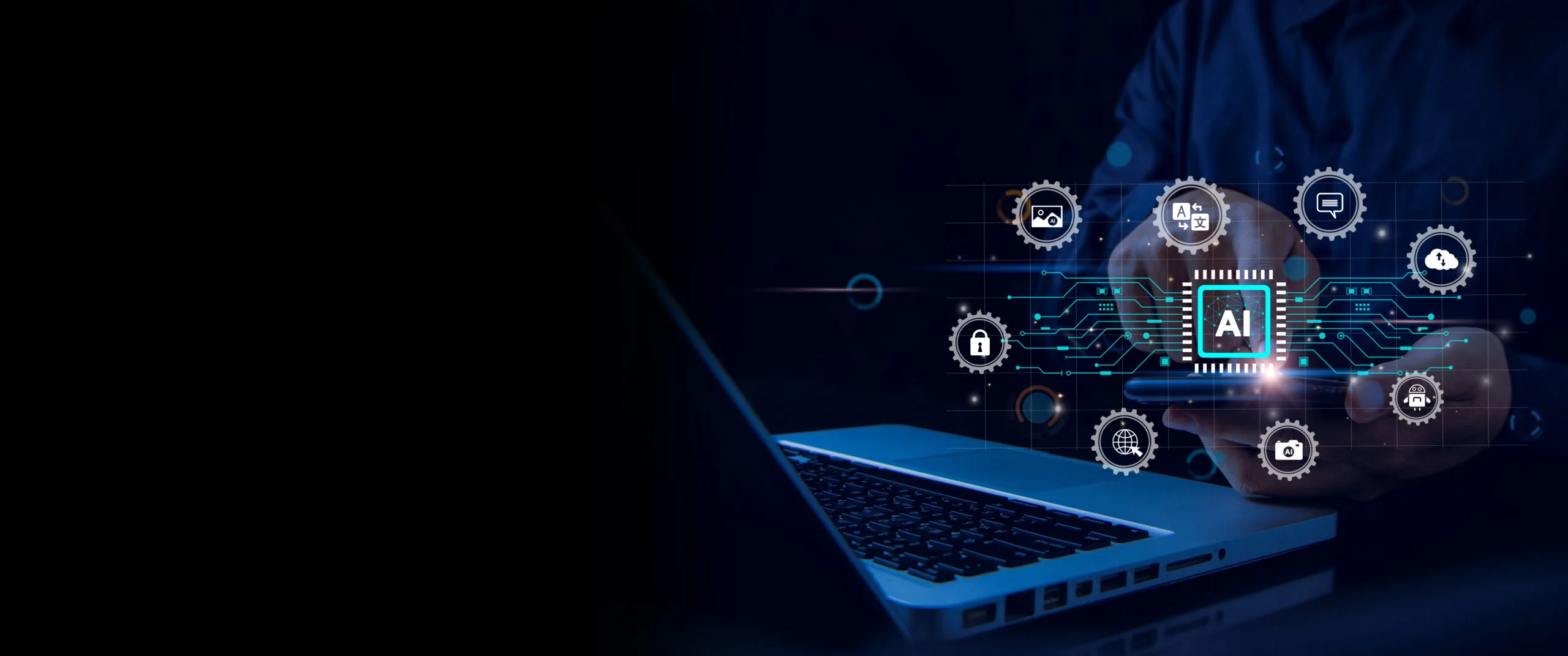
AI prompting guides for different job roles
Transform the way you work with our curated AI prompts, crafted for specific job roles to help you get the most out of your AI tools. Whether you’re looking to streamline tasks, spark creativity, or enhance productivity, our prompting guides offer instant support tailored to your needs. Download a prompting guide for your role today.